Background
Breeding forage grasses is complicated and the genetic gain is slow. Genomic selection will produce new improved and robust timothy cultivars more rapidly, making grassland-based agriculture more sustainable, and improve the competitive ability of Graminor cultivars. New knowledge on adaptation of timothy cultivars will enhance yield stability and make forage production more robust. This is especially important for the farmers in the northern Norway, where winter damages are of increasing concern. Seed companies will benefit by information on how to secure unique cultivar characteristics during seed multiplication of cultivars. Identification of more robust cultivars with better winter survival in a changing climate will increase the persistence of short-term leys and meadows. This will reduce the need for re-establishing swards due to winter damage, with greatly reduced carbon emissions from swards as the result. The project supports several of the UN sustainable development goals.
Objective
Main objective: To provide necessary knowledge and tools for more efficient and secure breeding and multiplication of timothy cultivars, especially for northern Norway.
Subgoals:
1) Clarify the risk for genetic shifts, especially in characters related to winter hardiness, and thus develop guidelines for safe, yet efficient seed multiplication of synthetic timothy cultivars and other open-pollinated forage grasses for northern areas.
2) Generate a draft genome of timothy using long-read sequencing technologies and advanced bioinformatics pipelines.
3) Use genotyping by sequencing (GBS) to develop SNP markers for timothy molecular breeding and tracking of allele frequency changes across generations.
4) Study differential expression of genes involved in frost and ice-encasement tolerance by RNA sequencing.
5) Develop novel prediction models by implementing historical climate data, soil data, and phenotype data using advanced machine learning models for developing robust cultivars.
Participants
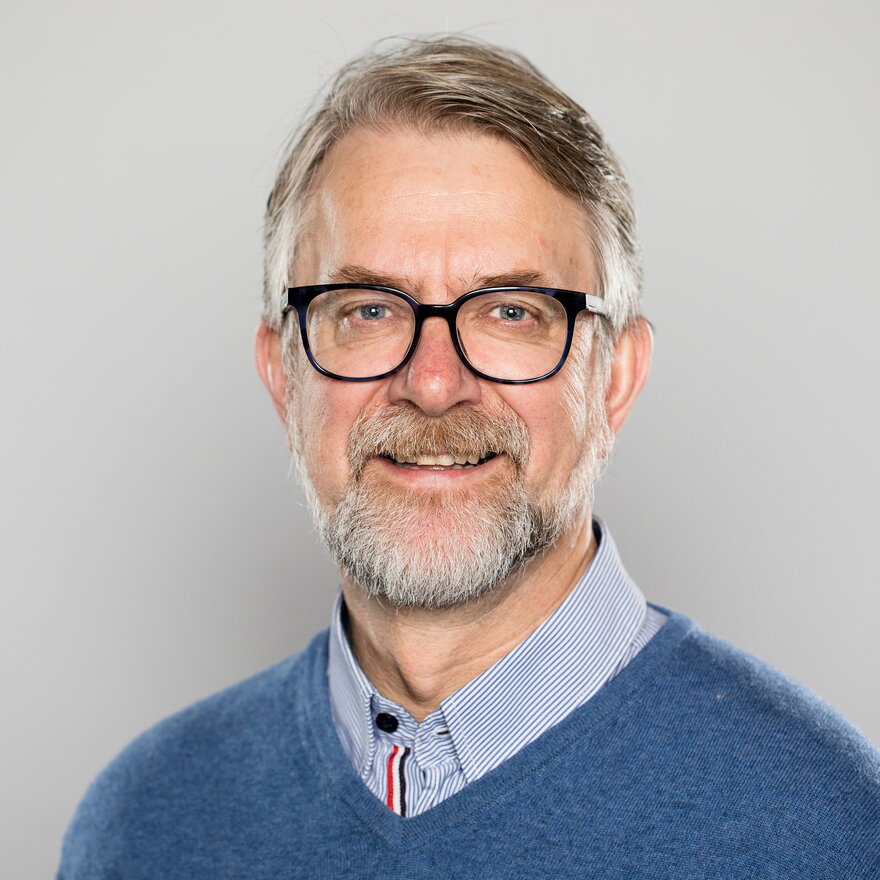
Odd Arne Rognli
Professor emeritus
Project leader
External participants
Muath Alsheikh
R&D Leader & Breeder, Graminor
Helga Amdahl
Breeder (Forage Crops), Graminor
Susanne Windju
Molecular Breeder, Graminor
Kristin Håland Gylstrøm
Assistant Breeder (Forage Crops) Graminor
Sigridur Dalmannsdottir
Research Scientist, NIBIO, Holt
Marit Jørgensen
NIBIO, Holt
Trygve S. Aamlid
Senior research Scientist, NIBIO, Landvik
Therese Mæland
Research Scientist, NIBIO, Særheim
Kristoffer Herland
Senior researcher, Norsk Regnesentral
Thordis L. Thorarinsdottir
Chief Research Scientist, Norsk Regnesentral