15 Dec 2023 - 31 Dec 2027
NFR (344288)
Kompetanse- og samarbeidsprosjekt / Samarbeid
FFL-JA-Forskningsmidlene for jordbruk og matindustri
About the project
- Grassland-based forage production plays a critical role in Norwegian agriculture for producing milk and meat and is crucial for the farm economy. With increasing temperatures due to climate change, perennial ryegrass, which is a high yielding and nutritious grass species, can be grown further north and in more continental regions. Expansion of the cultivation area of perennial ryegrass, which is mostly grown in monoculture for leys and requires high rates of nitrogen fertilization, leads to increased GHG emissions, and nitrogen leaching.
- To address this challenge, it is crucial to breed future perennial ryegrass cultivars with a higher nitrogen use efficiency (NUE). To speed up the development of improved cultivars, Norwegian perennial ryegrass breeding must be more efficient by incorporating advanced breeding methods and techniques.
- This project will utilize ecotypes and modern cultivars characterized in a previous Nordic/Baltic “Public-Private-Partnership (PPP) project for pre-breeding in perennial ryegrass”. NUE of these populations will be evaluated at two locations in Norway by integrating phenomics and genomics, to select the best NUE populations to incorporate in the breeding program. Phenomics creates huge amounts of data from various field sensors by drones and robots.
- Currently, no platform can process such big data in real-time. This project aims to establish an AI/ML-based data analytics platform for autonomous processing of big data, creating a real-time reporting application for dry matter yield and forage quality in grasses assisting breeders with rapid selection of superior genotypes and farmers with optimal harvest time decisions. The project will develop knowledge for breeding perennial ryegrass cultivars with improved forage quality and lower environmental footprints. Precision farming aided by utilization of the AI-based big-data platform will increase the economic output for farmers.
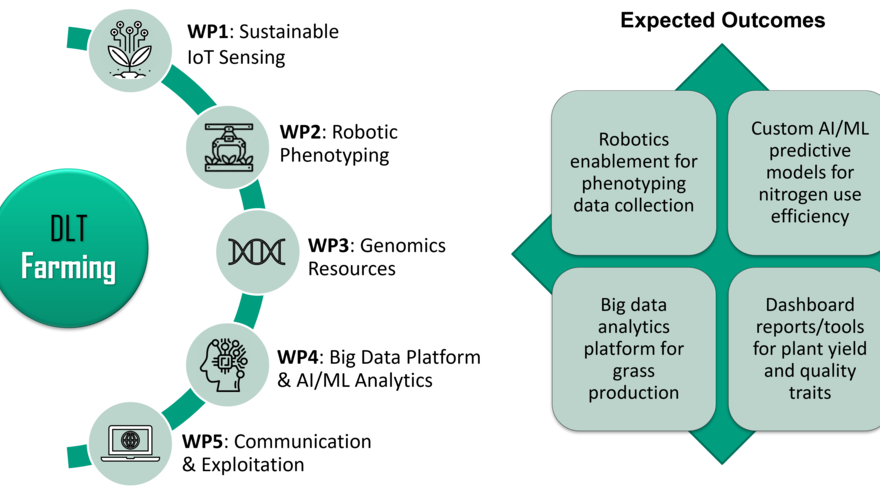
Background
Objectives
Participants